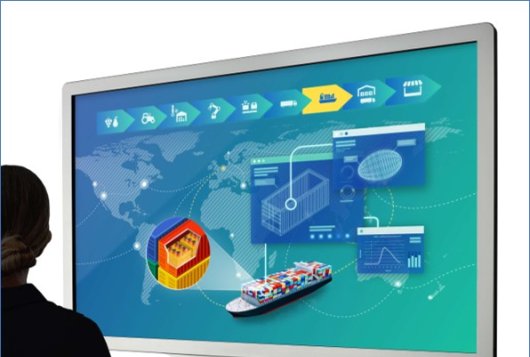
Longread
Digital Twins and Innovative Twins in Supply Chains
In 2019, WFBR was asked to write a paper about the digital developments in the Agro-Horti-Water-Food domain (in short the agrifood domain) [1]. The reason was that Smart Technology, the technology that connects the real world with the digital world, is foreseen to be a huge factor in getting the agrifood domain future proof. Food security, climate change mitigation, and sustainable use of raw materials are examples of the big challenges facing all food systems. And remaining profitable in these changing times, is and will continue to be the challenge for all actors in the supply chain.
Since 2019, the world has changed significantly. The Covid pandemic has happened. Serious conflicts have arisen in the world. Logistics have been disrupted. Energy and raw material costs have increased dramatically and became scarce.
At the same time, the Smart Technology world has not stopped developing. You can find news items about ChatGPT and Dall-e, two intelligent agents that create texts and illustrations that could easily have been made by humans [2]. You can read about autonomous vehicles, robots, and applications where intelligent agents, based on artificial intelligent developments, take over tasks of humans and do this with uncanny accuracy.
The key developments in Smart Tech for the agrifood domain in our view are (i) the availability of more and more advanced sensors and the resulting big data (ii) the rise of Artificial Intelligence software, and (iii) the development of digital twins and decision support tools. These developments come together in the area of Digital Twins, which will be the focus of this longread.
Sensors everywhere
The Dutch have a great saying: ‘meten is weten’, measuring is knowing. By measuring growth conditions, an indication of the initial quality of a fresh product can be obtained. By monitoring supply chain conditions such as temperature, humidity, gas conditions, by non-destructively determining quality aspects of the produce, by measuring fungal activity in the supply chain locations, an indication can be given on the current product quality and hence on the remaining shelf life. This means that supply chain decisions can be made based on the actual quality of the product batch travelling through the chain. And that local operations can be optimised because the sensors give precise insights of what is actually happening on location.
Two developments in sensor development are worthwhile to mention. First, many sensor developments move in a direction where the sensors get smaller, cheaper, and easier to use. They may become handheld, or integrated in a smart phone. They may operate on their own by having their own power source and internet connection. Examples of such sensors are temperature sensors, air humidity sensors, or O2 and CO2 sensors.

Two examples of sensors that are easy to use in practice.
Second, sensors are being developed that are robust for transportation with the product through the supply chain. We highlight here two examples. The first is a biokinetic fruit [3]. This is an artificial fruit sensor made in the shape of a fruit, with a material that mimics the internal temperature behaviour of the fruit. This way, the sensor can be used to accurately measure the product temperature profile of a batch of fruits during transport. The second is the AirSampler [4]. This is a box-shaped sensor in which every day during transport, a sample of the air in the reefer container is taken and stored for later analysis. This way, the ethylene profile of the cargo can be reconstructed. Both sensors help in determining what is really going on during transport, which helps in making better supply chain decisions.
In all cases, sensors gather data concerning a part of the supply chain. Put together, these data form a more complete picture of the fresh supply chain and the (quality) status of the product that travels through it.
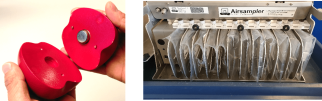
Two examples of in-transport measurements of supply chain conditions. On the left a biokinetic apple with a product temperature sensor inside. On the right the Airsampler after a trip of 19 days (19 filled bags with captured reefer container air)
Digital twins and decision support
With sensors generating data, and AI available to interpret these data, the road towards the development and use of digital twins [5] is open. Digital twins are a two or three stage system in which the real world is represented as data and information in the information world. The digital model of the real world can be used to suggest improvements, which can be tested and validated in the innovation world before being implemented on full scale. Together, the real (first stage), information (second stage) and innovation (whird stage) world form a three-stage dynamic system (see picture). The difference between a digital twin and a simulation is that the digital twin is actually connected to the real world through a (near) real time interface. The status of the digital representation of the supply chain and its fresh products is nearly exactly the same as the status of the real supply chain.
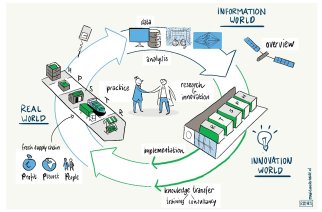
Artist impression of the three-stage innovation world created for WFBR by Ronald van
der Heide.
The powerful and interesting thing about digital twins of the supply chain is that they have access to data from many shipments of products that have been going through the supply chain. This allows for two levels of decision making:
First on the supply chain actions for the specific batch under consideration. Because of the detailed and real-time sensor information, the factors that influence aspects such as shelf life development (ripeness, firmness,…) and disease development (rot, chilling injury,…) can be accurately monitored. This allows the supply chain operators to e.g. decide on a logistics path that better matches the customer quality demand with the actual (or predicted) quality of the fresh product.
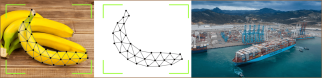
As an example of this direct level of decision making, we can consider a batch of bananas that is en route from South America to the Netherlands by ship. Chilling injury, skin splitting, premature ripening, cigar end rot or crown rot [6] may occur in such shipments. By measuring the air temperature, air humidity, and ethylene production during the various stages of the supply chain, and by adding these measurements together with banana data in an AI model, the occurrence of the various disorders and diseases can be predicted accurately, and the product owner may decide to unload the cargo in an earlier port, or let the bananas be shipped all the way to the end port.
Second, with the available information of numerous batches, it is possible to perform a pattern analysis of the supply chain performance in general. Can specific patterns be identified that are related to the different seasons, the different harvest moments? Can we say something about the relation between supply chain conditions, initial product quality and mould development? Are there supply chain improvements that would deliver 5% better product quality, 5% less energy usage, or 5% less greenhouse gas emissions?
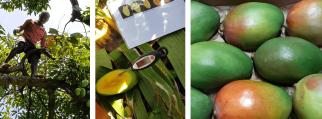
As an example of this pattern analysis level for decision making, we can consider the study for the effect of pre-harvest factors on postharvest mango quality as performed in the GreenCHAINge study [7]. By creating a large data set of mangoes over various years, with origins from different orchards where different chemicals were used. Sixteen pre-harvest treatments were used on the mangoes and the quality after shipping was determined in the research lab. No straightforward correlations could be identified between pre- and postharvest. However, from the dataset it became clear that temperatures between harvest and start of the transfer were very different: on some occasions mangoes remained warm for more than 48 hours before being pre-cooled and transported. This has a negative effect on mango quality and based on this insight, the conclusion was made that extra attention for this procedure was needed.
The role of the third stage: the innovation world
Digital twins, especially when coupled with the innovation world, the third stage, are a powerful tool for optimising supply chain operations. They can be used to address a brought range of challenges: the CO2 policies adopted by national governments and the EU (i.e. ships with lower sailing speed to reduce CO2 emissions, hence increasing the transit time for transport of fresh products), the high energy prices and lack of energy supply at peak moments (i.e. when energy shortages occur, consumers have priority over cold storage; this has impact on product quality), pesticide and fungicide restrictions as consequence of the Green Deal regulations of the EU (decrease of the chemical toolbox leads to fruits that are more susceptible to disease developments), among others. By working together in an optimal way, understanding what the product needs of the supply chain, and allowing for well-validated scenario studies, the effects of these transitions on product quality can be minimised.
When the digital twin is used to design supply chain improvements for implementation in the real world, it is wise (i) to match these proposals from the AI software with scientific knowledge and understanding of practical implications, (ii) to test these on a smaller scale under controlled circumstances, and (iii) to allow a proper analysis of the impact of the proposed change on the important economical and societal parameters for the chain under consideration.
This three-stage digital twin, also known as emulated twin, is in elements already in place in the form of research projects with the aim to optimize parts of the supply chain. When in such projects sensors are used to gather information, AI is used to perform the analysis, and research facilities are used to test, validate, finetune, accept or reject adjustments to one or more supply chain operations, there is in fact a rudimentary form of an emulated twin at work.

As an example, let’s take the Seed Vigour of Stored Seeds project [8]. The aim of this work is to find a correlation between the properties of fresh seeds and their vigour (an important quality parameter) after a long storage time. In this project, seeds are measured non-destructively using the iXeed DataCollector before they are stored. An AI algorithm has been developed that predicts the quality of the seeds based on the measured properties. This gives signals to the seed storage of when a batch should be delivered. In the research lab, the seeds have been measured, the seeds have been artificially aged and planted, and the seedlings have been measured again. In this way, the AI predictions were tested and validated, and accepted.
So is it then just a matter of relabeling normal research activities as digital twin activities? Not at all. Research activities will always take place to improve parts of the supply chain, without them being digital twin activities.
The huge difference with ‘normal’ research work and the added value of the three-stage digital twin is that it uses the potential of smart technology to make the bigger picture emerge when real world, information world, and innovation world meet. Short innovation cycles, evidence-based guesses, AI strength coupled to scientific knowledge of the experts, eye for people, planet and profit; these are the promises of the three-stage digital twin.
Several digital twin projects are already taking place at our research facilities in Wageningen. We work on third-stage digital twins in projects such as Future Sensors & Digital Twins [9], Smart manufacturing for baking [10] and Smart (Straw)berry Chain [11].